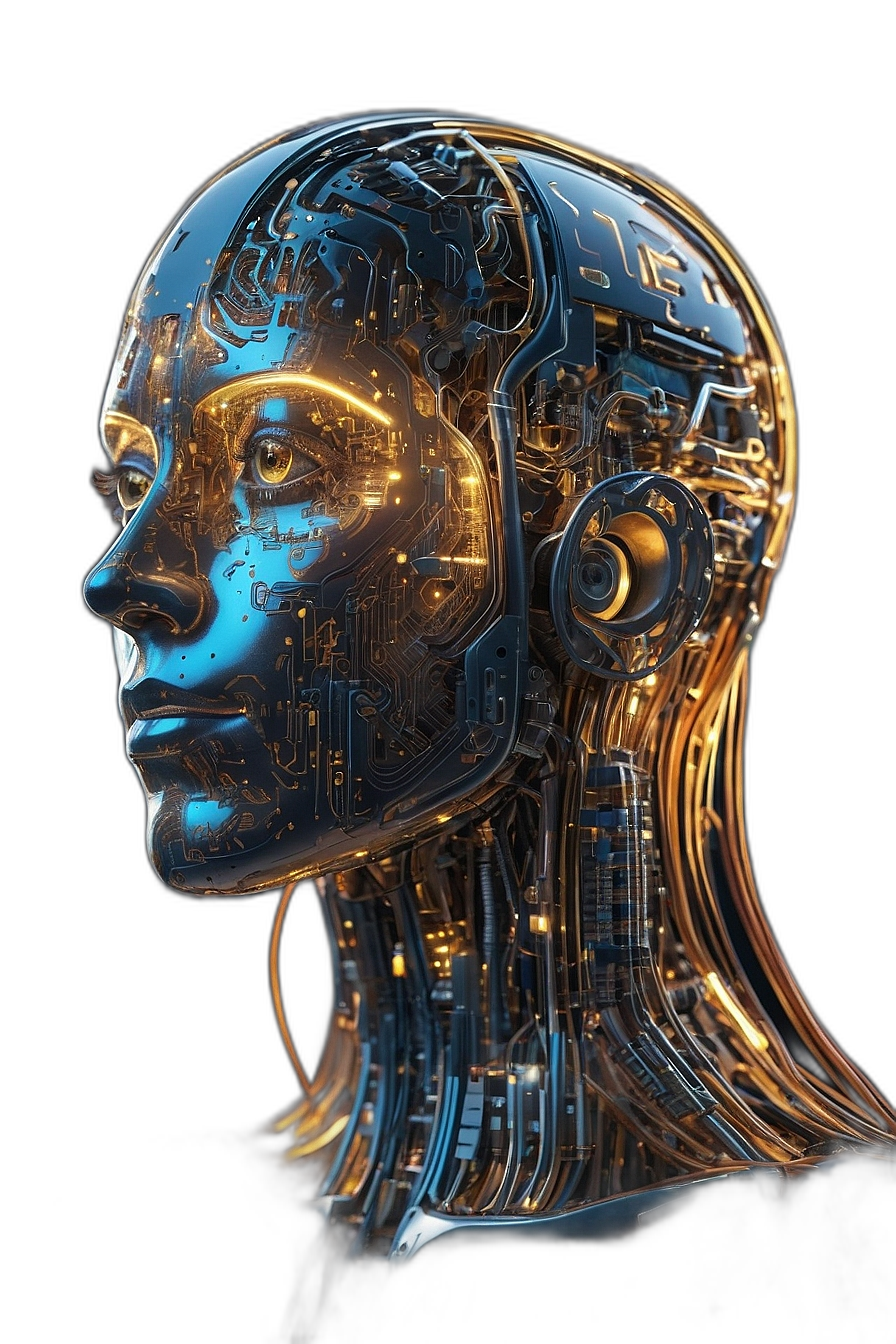
Prompt Engineering for OpenAI’s O1 and O3-mini Reasoning Models
Prompt Engineering for OpenAI’s O1 and O3-mini Reasoning Models
OpenAI’s O1 and O3-mini models are advanced systems designed for deep reasoning—meaning they “think” through problems much like a human would. Unlike the standard GPT-4 (sometimes called GPT-4o), these models are built to work through multiple steps internally without needing you to tell them to “think step by step.” Let’s break down how they differ from GPT-4o and discuss some best practices for designing prompts to get the best results.
Key Differences Between O1/O3-mini and GPT-4o
1. Input Structure and Context Handling
- Built-In Reasoning:
O1-series models come with an internal chain-of-thought. They naturally break down and analyze complex problems without extra nudges. GPT-4o, however, may need you to say things like “let’s think step by step” to work through multi-step problems. - Background Information Needs:
GPT-4o has a wide knowledge base and, in some cases, tools like browsing or plugins. In contrast, O1 and O3-mini have a more limited background on niche topics. This means if your task involves specific or less-common information, you need to include those details in your prompt. - Context Length:
O1 can handle up to 128,000 tokens and O3-mini up to 200,000 tokens (with up to 100,000 tokens in output). This is much more than GPT-4o, which allows you to include very detailed inputs—ideal for tasks like analyzing lengthy case files or large datasets.
2. Reasoning Capabilities and Logical Deduction
- Depth and Accuracy:
O1 and O3-mini are optimized for deep, multi-step reasoning. For instance, in complex math problems, O1 performed significantly better than GPT-4o because it naturally works through each step internally.- Complex Tasks: They excel in problems that require many steps (5 or more), producing highly accurate results.
- Simple Tasks: For very basic questions, their tendency to “overthink” can sometimes be a drawback compared to GPT-4o, which might give a quick, straightforward answer.
- Self-Checking:
O1 models internally verify their answers as they work, which often leads to fewer mistakes when handling tricky or multi-layered problems.
3. Response Characteristics and Speed
- Detail vs. Brevity:
Because they reason deeply, O1 and O3-mini tend to give detailed, step-by-step answers. If you prefer a concise answer, you need to instruct the model to be brief. - Performance Trade-offs:
- Speed and Cost: O1 is slower and more expensive because of its detailed reasoning process.
- O3-mini: Offers a good balance—it’s cheaper and faster while still strong in STEM tasks, though it might not be as strong in general knowledge as GPT-4o.
Best Practices for Prompt Engineering with O1 and O3-mini
To make the most of these models, here are some actionable tips:
Keep Your Prompts Clear and Direct
- Be Concise:
State your question or task clearly without extra words. For example, instead of writing a long explanation with lots of fluff, simply say:“Solve the following puzzle and explain your reasoning.”
- Minimal Context:
Only include necessary details. Overloading the prompt with too much extra information or multiple examples can actually confuse the model.
Use Few or No Examples
- Zero-Shot is Often Best:
Unlike earlier models that might need several examples to understand the task, O1 and O3-mini perform best with little to no examples. If you must include one, keep it extremely simple and relevant.
Set a Clear Role or Style with System Instructions
- Role Definition:
You can start with a short instruction like:“You are a legal analyst explaining a case step by step.”
This helps the model adopt the right tone and focus on the task. - Specify Output Format:
If you need your answer in a specific format (bullet points, a list, JSON, etc.), mention that in your prompt. For example:“Provide your answer as a list of key steps.”
Control the Level of Detail
- Directly Specify Verbosity:
Tell the model exactly how detailed you want the answer to be. For a short answer, say:“Answer in one paragraph.”
For a detailed breakdown, you could say:
“Explain all the steps in detail.” - Use Reasoning Effort Settings (for O3-mini):
If your interface allows it, adjust the reasoning effort (low/medium/high) based on how complex your task is.
Ensure Accuracy in Complex Tasks
- Provide Clear Data:
If your task includes numbers or specific facts (like in a legal case), structure them clearly. Use bullet points or tables if necessary. - Ask for Self-Check When Needed:
For critical tasks, you might ask the model to double-check its work. For example:“Analyze the data and verify that your conclusion is consistent with the facts.”
- Iterate When Necessary:
If the answer isn’t quite right, try a slightly rephrased prompt. Running the prompt a few times and comparing results can increase confidence in the final answer.
Example: Applying These Practices to a Legal Case Analysis
Imagine you need a legal analysis using one of these models. Here’s how you might structure your prompt:
- Outline the Facts Clearly:
Begin with a list of the key facts. For example:“- Party A and Party B entered a contract on 2025.
- There was a disagreement about delivery dates.”
Then ask:
“Based on the above facts, determine if Party A is liable for breach of contract under U.S. law.”
- There was a disagreement about delivery dates.”
- Include Relevant Legal Context:
If the analysis depends on specific laws or precedents, include that text in the prompt.“According to [Statute X]: [insert excerpt]. Apply this statute to the case.”
- Set the Role and Format:
Provide a system instruction such as:“You are a legal analyst. Use the IRAC format (Issue, Rule, Analysis, Conclusion) in your response.”
- Control the Level of Detail:
Specify if you want a thorough explanation or a brief summary:“Explain your reasoning in detail, covering each step of the legal analysis.”
- Ask for Verification:
Finally, add:“Double-check that all facts are addressed and that your conclusion logically follows.”
By following these steps, you guide the model to produce a well-structured and accurate legal analysis.
Summary of Best Practices
- Be clear and concise: Focus on your main question and include only the necessary details.
- Limit examples: Use zero-shot or at most one simple example.
- Define roles and formats: Set the model’s persona and output style early on.
- Control verbosity: Directly instruct whether you want a brief or detailed response.
- Provide clear data: Structure any critical facts or data clearly.
- Verify critical outputs: Ask the model to double-check its reasoning for complex tasks.
Using these guidelines helps you tap into the powerful reasoning capabilities of O1 and O3-mini. They’re best for in-depth tasks like complex legal analysis, detailed problem solving in math, or other situations where a step-by-step breakdown is essential. For simpler queries, GPT-4o might be faster and more direct, so always choose the right tool for your task.
This plain-language rewrite covers all the ins and outs of prompt engineering for OpenAI’s advanced reasoning models, ensuring you have actionable insights to optimize your prompts for accurate and detailed responses.